In the ever-evolving landscape of technology, one term that's been gaining significant traction is "edge computing." This distributed computing paradigm is poised to revolutionize the way we process and analyze data, particularly for applications that demand low latency and high performance, such as real-time analytics and machine learning.
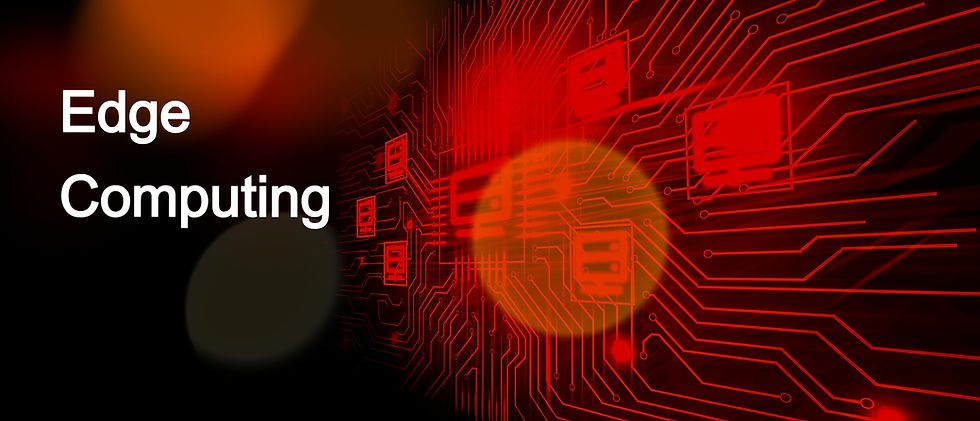
The Rise of Edge Computing
Traditionally, data processing has been centralized, with data traveling from endpoints to data centers or cloud servers for analysis and storage. While this approach has served us well, it has limitations, especially when it comes to applications that require immediate responses or operate in remote locations. Enter edge computing, which flips the script and takes computation and data storage closer to where data is generated and consumed.
Bringing Computation Closer to the Source
At its core, edge computing is about minimizing the physical distance that data needs to travel. Instead of sending data to a remote data center or cloud server, edge devices process and analyze data locally or within a nearby edge server. This shift significantly reduces latency, making it ideal for applications that demand real-time responsiveness.
Imagine a self-driving car that needs to make split-second decisions to navigate safely. In a traditional setup, sending data to a remote server for processing and waiting for a response could result in delays and potentially hazardous situations. With edge computing, the car's onboard computer can process data from its sensors in real-time, ensuring rapid decision-making and enhanced safety.
Real-time Analytics: A Game Changer
One of the most promising aspects of edge computing is its ability to facilitate real-time analytics. Businesses across various industries are increasingly reliant on instant insights from their data. Whether it's monitoring industrial machinery for maintenance needs, tracking customer behavior in retail stores, or ensuring the efficient operation of energy grids, real-time analytics can make a world of difference.
With edge computing, sensors and devices can analyze data on the spot, generating immediate insights. For example, a manufacturing plant can use edge devices to detect anomalies in its machinery and trigger maintenance alerts in real-time, preventing costly breakdowns and downtime.
Edge Computing and Machine Learning
Machine learning, with its data-hungry algorithms, also stands to benefit immensely from edge computing. Consider the use case of smart cameras in security systems or autonomous drones. These devices need to process large amounts of data (like images and videos) quickly to identify objects or patterns. Edge computing enables them to run machine learning algorithms locally, delivering faster results.
Moreover, edge computing allows for privacy-sensitive data to be processed locally, reducing the need to transmit sensitive information to external servers. This is crucial for applications like healthcare, where patient data security is paramount.
Conclusion
In a world where data-driven decision-making and real-time responsiveness are increasingly vital, edge computing is a game-changer. By bringing computation and data storage closer to the devices generating and consuming data, it reduces latency, improves performance, and opens up new possibilities for applications such as real-time analytics and machine learning.
As technology continues to advance, we can expect edge computing to play an increasingly prominent role in shaping the future of data processing and analysis. Embracing this paradigm shift could be the key to gaining a competitive edge in an era where speed and efficiency are paramount. Edge computing is not just a buzzword; it's a transformative force that's already making its mark on the world of technology.
Commentaires